One of the main aims of EPoCH is to understand whether parents’ lifestyles in the prenatal period causally affect the health of their children. The project will use several techniques to try to tease apart correlation from causation. In our first blog post, I’m going to try to explain why correlation does not equal causation and why we often need to separate the two.
Example: does drinking a small amount of alcohol every day reduce your chance of developing heart disease?
We might see in the news that a study finds that people who drink a small amount of alcohol every day have lower rates of coronary heart disease than people who don’t drink at all (in fact, here are a few examples of news articles along these lines). The article might then say that this means we can all reduce our risk of heart disease by enjoying a lovely drop of booze every day.
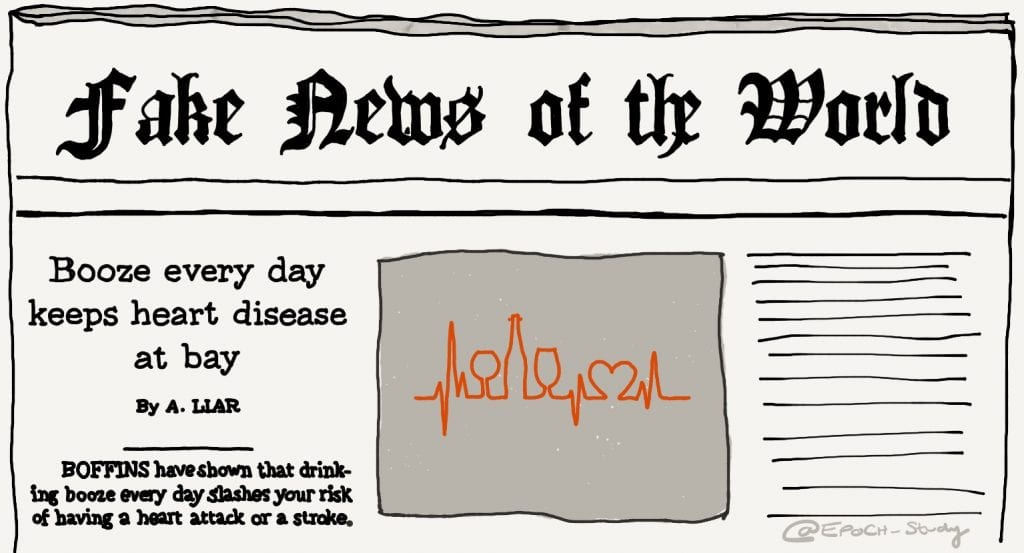
Observational evidence
More often than not, these sorts of studies are based on “observational evidence”, i.e. evidence that has been gathered by observing a group of people enrolled in either a “cohort” or a “case-control” study. There is no experiment, the researchers haven’t changed anything, and the people taking part in the study haven’t received any treatment or intervention. Instead, the researchers have compared the rate of heart disease in people who say they drink a little and people who say they don’t drink, and they have observed that, proportionally, more people who don’t drink have heart disease.

All of the studies used in EPoCH are observational studies. They are birth cohorts that enrol mothers, partners and children at the time of pregnancy or the child’s birth and then observe them over time (not in a creepy way, but with their full consent, using questionnaires and interviews, etc). So, it’s really important that we are aware of the different interpretations of observational evidence.
Potential interpretations of observational evidence
In the alcohol-heart disease example, let’s think of possible reasons why people who drink small amounts of alcohol might have a lower risk of heart disease:
1. Drinking small amounts of alcohol reduces the chance of getting heart disease.
This is the explanation the news article has homed in on (although, without further evidence, there is no reason to believe this explanation over the others below). It suggests that drinking alcohol causes lower risk of heart disease, i.e. there is a true causal effect from alcohol –> lower heart disease.
2. Having heart disease makes people give up drinking.

This isn’t as enticing at the first explanation, because it doesn’t mean we can all indulge in our penchant for Babycham on a nightly basis, but without further evidence, it is equally likely. In fact, given what we already know (from other studies) about alcohol generally being bad for us, we can assume that this explanation is more likely. Most people who drink probably change their drinking habits if they find out they’ve got heart disease. They might give up completely. They might even be in hospital, where alcohol is not available. This is an example of reverse causation, i.e. the direction of causal effect is from lower heart disease –> alcohol.
For EPoCH, where we’re interested in prenatal influences on childhood health, reverse causation is less likely. For example, a child’s IQ at age 7 can’t feasibly affect whether the child’s father drank coffee before they were born. In fancier words, the temporal order of events makes reverse causation impossible.
3. People who drink a small amounts of alcohol have something else about them that reduces their chance of getting heart disease, and it’s that thing (not the alcohol) that’s responsible for the link.
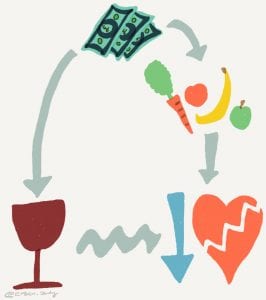
The “something else about them” could be anything, but say for example that people who drink small amounts of alcohol are wealthier than people who don’t. If this is true, then wealth affects both alcohol and risk of heart disease, thereby creating a false or a stronger relationship between those two factors. If we imagine that many of the people who are drinking a small amount of alcohol every day are middle class people drinking a glass of wine with their dinner, then we might imagine that these people can also afford a high quality diet, better access to health care and many of the other health advantages to having a higher socioeconomic position. So it makes sense that, in addition to being more likely to drink in moderation, wealthy people might be less likely to have heart disease. In this case, the relationship between alcohol and heart disease would be explained partly, or even completely, by the differences in wealth between the two groups. This is an example of “confounding” (where the confounder in this example is wealth), i.e. higher wealth –> alcohol, and, higher wealth –> lower heart disease, with no (or a weaker-than-estimated) direct causal effect between alcohol and heart disease in either direction.
4. Drinking small amounts of alcohol is not related to having a lower risk of heart disease in the general population, but it appears to be in this study because of bias.
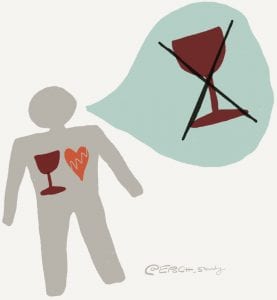
There are lots of types of bias that can distort the results of a study so that they don’t match up with what we would see if we had perfect data on the whole population. One example is reporting bias whereby participants in the study misreport their behaviour (for example, because they can’t remember, or because they feel uncomfortable reporting the truth). In this case, people with heart disease might say they don’t drink because they have been told by a doctor that they should limit their alcohol intake and they want to give the “right” answer, even though, in reality, they do drink. Another example is loss-to-follow-up bias whereby participants die or drop out of the study because of the thing being studied before all of their data can be collected. In this case, drinking a little may in fact cause people to either die or drop out of the study before they are recorded as having heart disease. Therefore the group that doesn’t drink will be left with a higher proportion of people with heart disease than the group that drinks small amounts. In both these examples, bias would distort the numbers to make it appear like people who don’t drink have a higher risk of heart disease than those that drink a small amount (i.e. the results of the observational study).
5. Drinking small amounts of alcohol is not related to having a lower risk of heart disease in the general population, but it appears to be in this study because of chance.
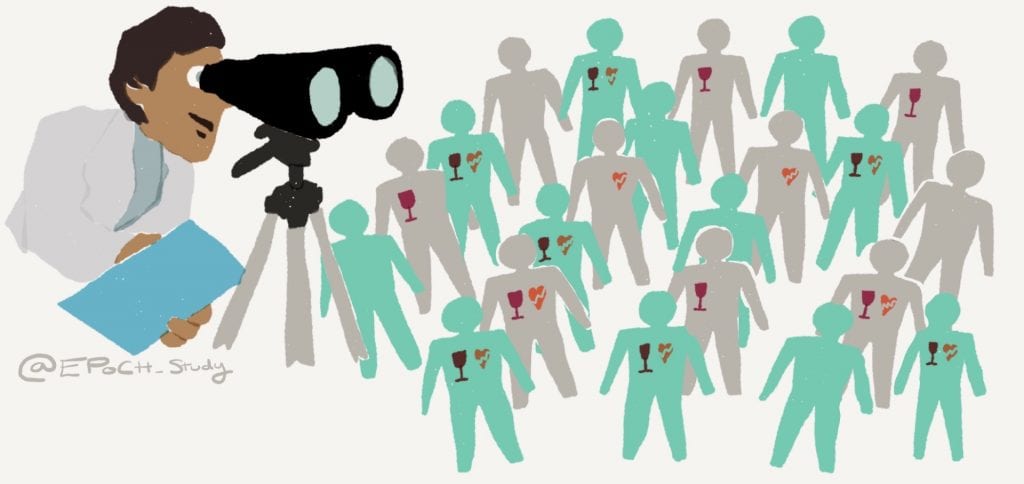
Because the study is based on just a sample of people, the researchers might have found a fluke association that they wouldn’t find if they were to re-run their study using a different sample, or using everyone in the population. The researchers will have tried to rule out this explanation by conducting statistical tests that give a measure of “precision” (i.e. P-values and confidence intervals), but chance findings can never be ruled out completely.
So correlation does not imply causation… but why do we care?
The news article’s claim that drinking alcohol can slash the risk of heart disease (i.e. the interpretation of the observational evidence as evidence of explanation 1 – a true causal effect) is a classic example of taking correlative evidence and making causative claims, i.e. confusing correlation and causation.
But why is it important to know whether something causes a disease or is merely correlated?
The clue is in the way the news article has framed the evidence, i.e. that we can all reduce our risk of heart disease by drinking alcohol every day. Identifying causal relationships helps us identify things we can modify to improve our health. If there was a lot of strong causal evidence that drinking a small amount every day really does reduce our risk of heart disease, then doctors would start prescribing alcohol.
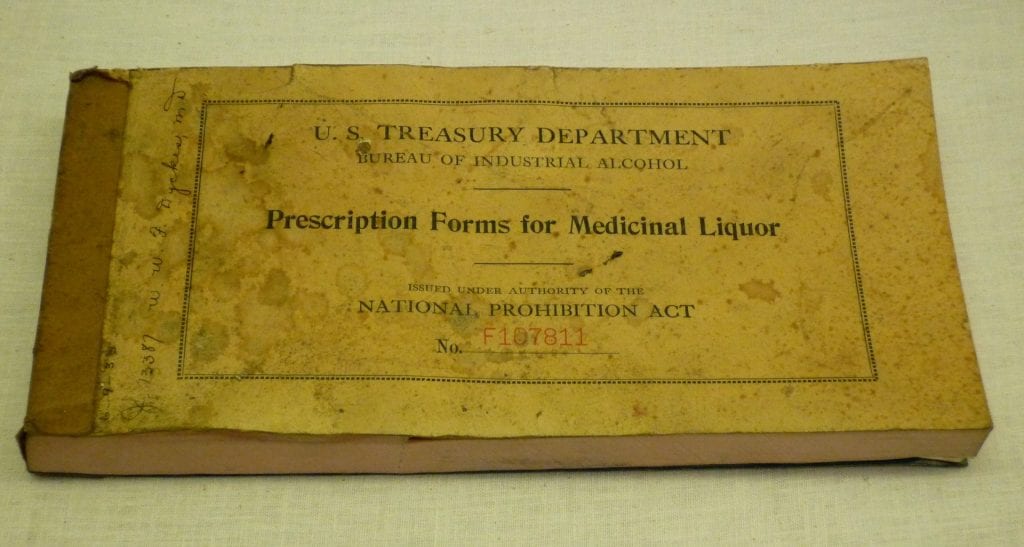
What will EPoCH do?
A lot of the current health advice given to parents (mostly mums) is based on correlative rather than causative findings from observational studies, so we desperately need better, causal evidence to support this advice.
In EPoCH, we’ll use statistical techniques to try to tease apart correlation from causation. We’ll also work with the media and groups like WRISK to help make sure our findings are interpreted accurately by journalists, policy makers, healthcare professionals and ultimately parents.
You can find out more about our plans, methods and results by exploring our blog.